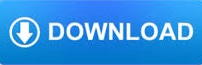

The availability of flowCore and flowViz has enabled the creation of new tools for quality assessment of large FCM experiments, such as flowQ, and for model-based clustering and automated gating, such as flowClust. The Bioconductor model for FCM data analysis facilitates the development of new analysis methods, since the overhead associated with accessing and visualizing FCM data is handled by flowCore and flowViz. The basic data processing tools for importing, transforming, gating, and organizing raw FCM data are in the flowCore package and the visualization functions are in flowViz. It is therefore desirable to develop programmatic approaches to process FCM data so that FC-HCS analysis pipelines are robust, objective, and able to match the high-throughput capacity of modern cytometers.įCM packages available through the Bioconductor project provide an open platform that can be used by cytometrists, bioinformaticians, and statisticians to collaboratively develop new methods for automated FC-HCS analysis.
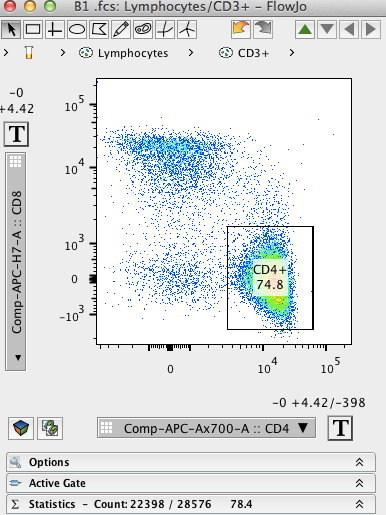
#HOW TO CHANGE DASHED LINE IN FLOWJO 10 MANUAL#
Unfortunately, this approach to FC-HCS analysis results in methods that are semiautomated at best, and they often require significant subjective and error-prone manual intervention to identify cells of interest. Results are then exported, and statistical analysis is performed in packages like MATLAB (USA) and R ( ). The raw FCM files are processed and gated using FCM specific software, such as FlowJo or FCS Express (De Novo Software, USA).
#HOW TO CHANGE DASHED LINE IN FLOWJO 10 SOFTWARE#
Current FC-HCS data analysis methods often use a combination of software packages for different parts of the analysis.

Flow Cytometry-High-Content Screening (FC-HCS) experiments generate large volumes of data, which requires a systematic approach to preprocessing, gating (i.e., filtering), and summarizing results for robust analyses. While there are a number of different software packages available for analysis of FCM data, these programs are often ill-suited to the development of new methods needed for analyzing high-throughput FCM studies. We show that the expression values for markers characterized using the automated approach in plateCore are in good agreement with those from FlowJo, and that using plateCore allows for more reproducible analyses of FCM screening data. This same data set was also manually analyzed by a cytometry expert using the FlowJo data analysis software package (TreeStar, USA). plateCore was used to analyze data from a BD FACS CAP screening experiment where five Peripheral Blood Mononucleocyte Cell (PBMC) samples were assayed for 189 different human cell surface markers. We created plateCore, a new package that extends the functionality in these core packages to enable automated negative control-based gating and make the processing and analysis of plate-based data sets from high-throughput FCM screening experiments easier. Flow cytometry (FCM) software packages from R/Bioconductor, such as flowCore and flowViz, serve as an open platform for development of new analysis tools and methods.
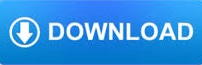